Achievement
Evaluating machine-generated text
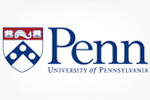
Project
The Dynamics of Communication in Context
University
University of Pennsylvania
(Philadelphia, PA)
PI
Research Achievements
Evaluating machine-generated text
IGERT Trainee Emily Pitler and her advisor Ani Nenkova (Computer Science) have been developing automatic methods for evaluating the quality of machine-generated text. Humans effortlessly produce novel utterances which are grammatical, factual, and relevant. In contrast, machine-generated texts often are ungrammatical, incoherent, or redundant. The ability to generate more natural text would greatly improve several applications of natural language processing, such as language translation, summarization, and question answering. An important first step in producing more fluent text is the ability to measure how fluent a machine generated text is. Using human evaluations of human-generated and machine-generated text, they have been able to develop a system that can evaluate both the syntactic and referential coherence of new, previously unseen text. This method will allow for significant breakthroughs in developing natural language computer systems.
- “Research Achievements”
- Achievements for this Project