Achievement
Computational models of learning in the brain
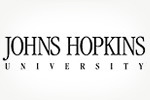
Project
IGERT: Unifying the Science of Language
University
Johns Hopkins University
(Baltimore, MD)
PI
Trainee Achievements
Computational models of learning in the brain
Computational models of learning in the brain have differentiated networks for rapid storage of individual experiences and networks for extracting the regularities characterizing a cognitive domain. The former is hypothesized to characterize the hippocampus, the latter, cerebral cortex. Trainee Teresa Schubert, working with Prof. Brenda Rapp, tested this theory by training participants to learn the spelling of pseudo-words in an MRI scanner. As the words were learned, activity in the hippocampus, but not the cortex, correlated with memory strength, as inferred from error patterns during learning. During learning, activity in the cortex, but not the hippocampus, correlated with numbers of learning trials on a given word. This is expected under the theory: the hippocampus, but not the cortex, is rapidly accumulating increasingly strong memory traces of each word, while the cortex is processing each word and thus displaying the gradual activity decrease indicative of repeated processing.
SEE MORE:
- “Trainee Achievements”
- Achievements for this Project